Online jewelry retailers face a distinct challenge - customers typically make purchasing decisions based on the appearance of a piece of jewelry. This makes it critical for these stores to provide visually appealing and accurate product recommendations to their online customers.
In this article, we will showcase how a chain of jewelry stores leveraged Kea Labs Visual recommendations to improve their online recommendations and drive sales.
However, the demand for visually similar product recommendations is not unique to this particular jewelry store. In fact, many other jewelry stores struggle with providing accurate and visually appealing product recommendations, which can ultimately hurt their online sales.
For this specific jewelry store, the challenges were even greater. The store offers approximately 26,000 products, ranging from affordable prices to exclusive jewelry. The service needed to be able to identify a customer's preferred price point and take it into account when making product recommendations. The store also regularly holds promotions, such as holiday sales and discounts on specific products, that needed to be taken into consideration when providing recommendations. In addition, the speed of delivery was influenced by various factors, such as product availability in different stores, warehouses, and supplier warehouses, and whether the product was made to order. All of these factors needed to be considered when making recommendations to ensure that the customer had an accurate and positive experience with the store.
To address the challenges outlined above, the jewelry store decided to use two Kea Labs products: advanced product recommendations with personalization and visual recommendations.
First, the most in-demand blocks were added to the product page. These blocks included visually similar products, similar products, and products from collections:
In this article, we will showcase how a chain of jewelry stores leveraged Kea Labs Visual recommendations to improve their online recommendations and drive sales.
However, the demand for visually similar product recommendations is not unique to this particular jewelry store. In fact, many other jewelry stores struggle with providing accurate and visually appealing product recommendations, which can ultimately hurt their online sales.
For this specific jewelry store, the challenges were even greater. The store offers approximately 26,000 products, ranging from affordable prices to exclusive jewelry. The service needed to be able to identify a customer's preferred price point and take it into account when making product recommendations. The store also regularly holds promotions, such as holiday sales and discounts on specific products, that needed to be taken into consideration when providing recommendations. In addition, the speed of delivery was influenced by various factors, such as product availability in different stores, warehouses, and supplier warehouses, and whether the product was made to order. All of these factors needed to be considered when making recommendations to ensure that the customer had an accurate and positive experience with the store.
To address the challenges outlined above, the jewelry store decided to use two Kea Labs products: advanced product recommendations with personalization and visual recommendations.
First, the most in-demand blocks were added to the product page. These blocks included visually similar products, similar products, and products from collections:
- The visually similar products block was configured to find products that were visually similar to the current product, while also taking into account the business rules and product characteristics such as metal, main stone, color of the main stone, and occasion for purchase (such as engagement, wedding, birthday, etc.).
- The similar products block was focused on finding other products in the same price range, with similar characteristics and occasions, but not necessarily visually similar.
- The products from collections block was designed to detect and show products that belonged to the same collection or set.
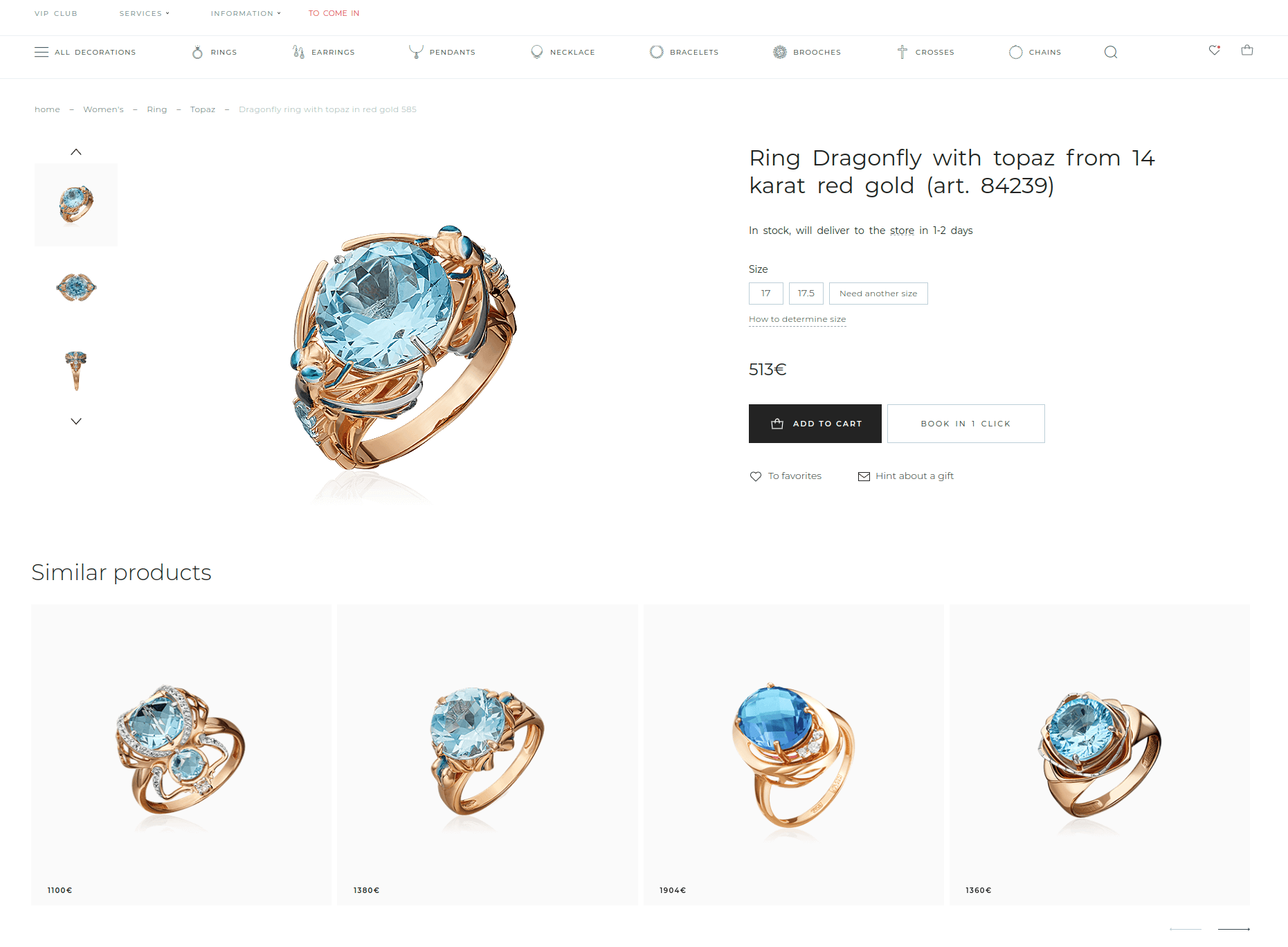
On other pages, personalization based on visitors' visual preferences was used to create a personalized product recommendation. This algorithm takes into account the visual features of the viewed products, identifies the interests of the visitor, and forms a personalized selection of products. In an A/B/C test against three other personalization algorithms, the visual personalization algorithm proved to be the most effective, significantly outperforming classic algorithms such as Collaborative Filtering. We will cover this case in another article.
Additionally, to ensure that the recommendations were always up-to-date, an API was created to synchronize product data in real-time between Kea Labs and the store's inventory management system. The system was set up to receive regular updates on product availability, promotions, and discounts, which were then used to create personalized recommendations and adjust the visual similarity algorithm. This allowed the store to ensure that the recommended products were always in stock, and that the latest promotions and discounts were reflected in the recommendations.
Overall, the implementation of Kea Labs' advanced product recommendations with personalization and visual recommendations helped the jewelry store to address its challenges, and significantly improve the customer experience on its website.
Additionally, to ensure that the recommendations were always up-to-date, an API was created to synchronize product data in real-time between Kea Labs and the store's inventory management system. The system was set up to receive regular updates on product availability, promotions, and discounts, which were then used to create personalized recommendations and adjust the visual similarity algorithm. This allowed the store to ensure that the recommended products were always in stock, and that the latest promotions and discounts were reflected in the recommendations.
Overall, the implementation of Kea Labs' advanced product recommendations with personalization and visual recommendations helped the jewelry store to address its challenges, and significantly improve the customer experience on its website.
- After implementing Kea Labs' visual recommendation solution, the jewelry store saw a significant improvement in their recommendation strategy. The visually similar product block achieved an impressive 40% click-through rate, indicating that visitors were finding the recommendations to be relevant and useful.
- This resulted in increased page views and engagement, with visitors who used the recommendations viewing 2.5 times more products than other engagement visitors.
- Additionally, the store analyzed online purchases, requests, and customer inquiries, and found that at least half of the visitors who took target actions, such as making a purchase, used Kea Labs' recommendation system to discover new products. Furthermore, approximately one-third of the online purchases were from products that were first viewed in the visual recommendations block.
- As a result of these positive outcomes, the store made the decision to replace their search engine with Kea Labs' search solution, which provided a more complete and personalized user experience. This allowed the store to meet their customers' needs more effectively and streamline the overall shopping experience.
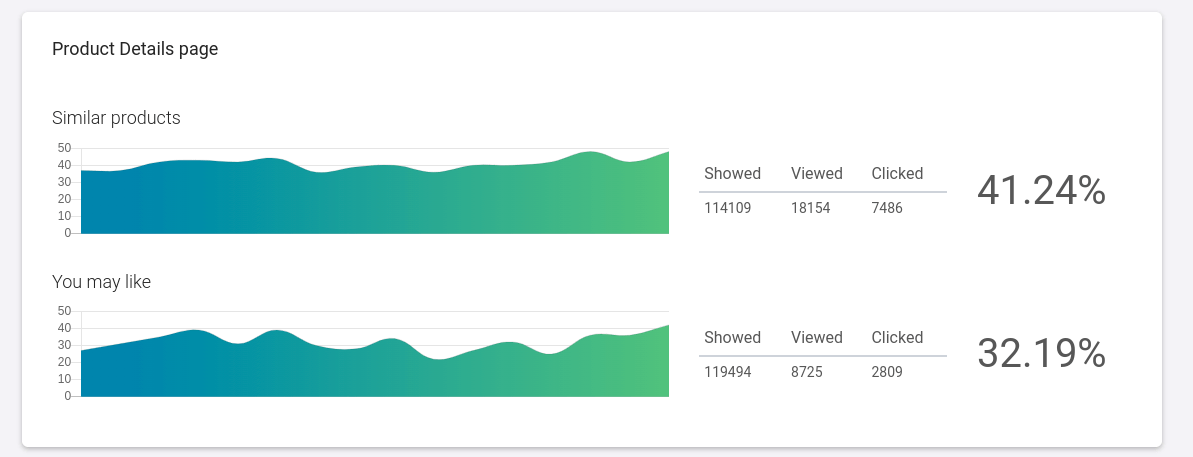
Conclusion:
The jewelry industry is highly competitive, and it can be challenging to stand out in a crowded market. By leveraging Kea Labs' advanced AI solutions, this store was able to overcome the unique challenges they faced and provide their customers with a more personalized and visually appealing shopping experience. The results speak for themselves, with a significant increase in engagement and sales that directly impacted the store's bottom line.
By adopting Kea Labs' visual recommendation solution, this jewelry store was able to differentiate themselves in a crowded market, increase customer satisfaction, and drive sales. This case study demonstrates the value of implementing AI-driven solutions to improve the overall customer experience and gain a competitive edge in the market.
The jewelry industry is highly competitive, and it can be challenging to stand out in a crowded market. By leveraging Kea Labs' advanced AI solutions, this store was able to overcome the unique challenges they faced and provide their customers with a more personalized and visually appealing shopping experience. The results speak for themselves, with a significant increase in engagement and sales that directly impacted the store's bottom line.
By adopting Kea Labs' visual recommendation solution, this jewelry store was able to differentiate themselves in a crowded market, increase customer satisfaction, and drive sales. This case study demonstrates the value of implementing AI-driven solutions to improve the overall customer experience and gain a competitive edge in the market.